AI Futures: How AI is augmenting Singapore's healthcare
By Amanda Oon
Interview with Dr Goh Han Leong, Principal Specialist, Data Analytics & Ai, IHiS.
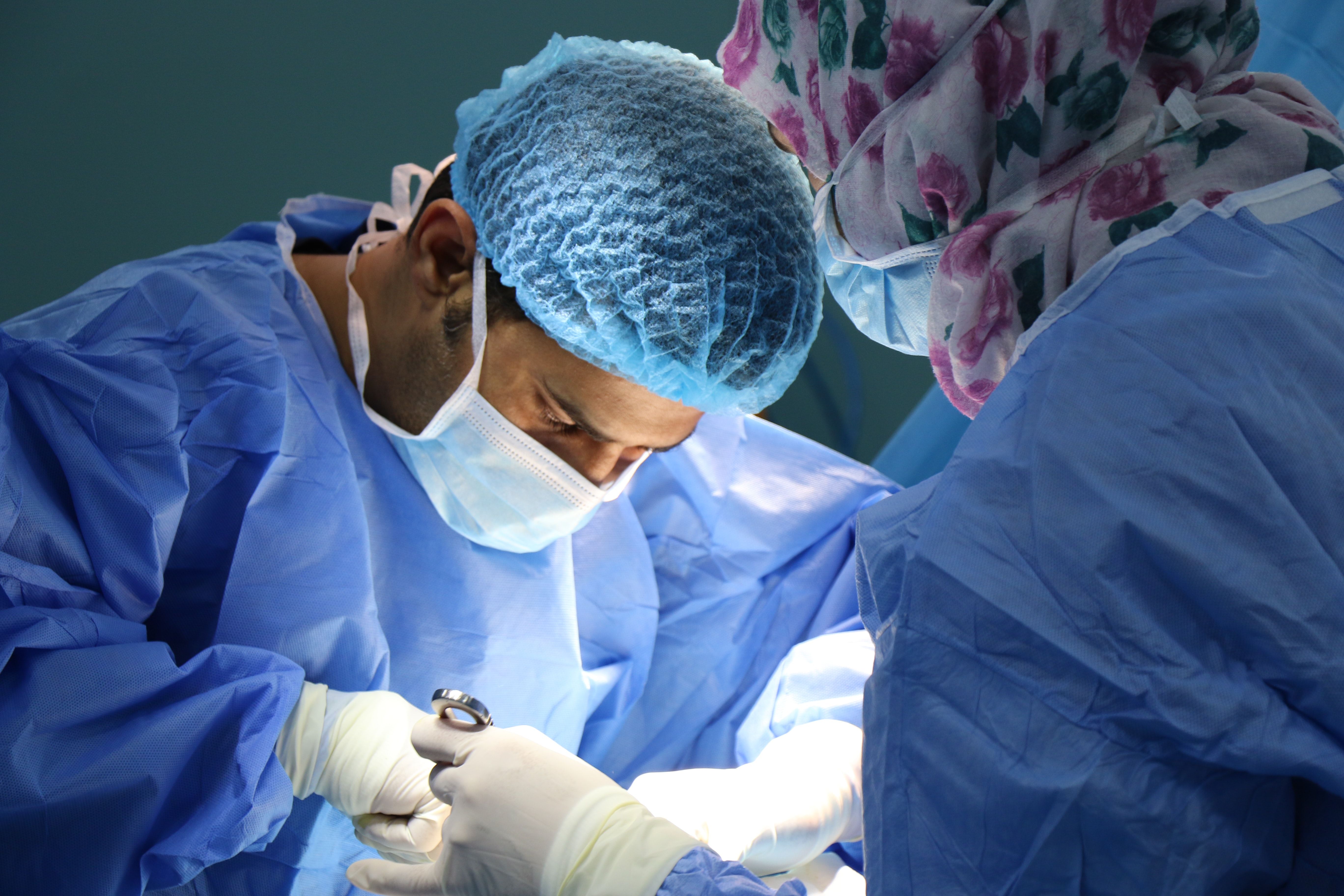
Singapore has done good work in leveraging data to enhance health and healthcare operations. Some notable examples include the National Electronic Health Record (NEHR) that securely collects summarised patient health records across different healthcare providers.
This “one patient, one health record” approach ultimately enables patients to receive more seamless care, and allows healthcare professionals to deliver safer and more personalised care to patients. An added benefit is that it also gives hospital administrators and policy makers a holistic view of our population health, and facilitates more efficient and coordinated patient care across healthcare institutions.
Another example is the Command, Control, and Communication Systems (C3) that harnesses data to give a bird’s eye view of thousands of ongoing operations indicators at Tan Tock Seng Hospital, with real-time visibility of patient flow and resources on the ground.
The next big thing is probably digital phenotyping in which data generated passively by mobile devices, such as smartphones, can be collected with patient consent for a broad range of potential clinical uses (e.g. stress identification, management of chronic diseases, early detection of dementia). Integrating a person’s digital signals with their healthcare utilisation can circumvent the restriction of a one-size-fits-all treatment of diseases and provide more proactive health interventions to the patient.
Having said that, we should open the data door with caution. Besides societal considerations like data privacy, we must be mindful of not getting lost in data noise as technology makes more and more different types of healthcare data available.
Poor data quality or understanding of the data collected will result in a garbage-data-in and garbage-insights-out situation. To respond to the data opportunities, we should also invest in building a trusted shared platform that has good data provenance and lineage. In addition, a strong AI capability would be good to auto-encode/harmonise the data for a unified view of different data.
How can AI help improve and provide better patient care? How can it help improve the role of hospital and healthcare professionals?
My view is AI has been useful in silos at different stages of healthcare services such as Population Screening, Early Detection & Diagnosis, and Treatment & Disease Management.
For example, in Population Screening, we have the Singapore Eye LEsioN Analyzer (SELENA+), deployed by the Singapore National Eyecare Centre and supported by IHiS. SELENA+ is an AI-powered application that grades eye scans, cutting the time and effort needed to spot signs of diabetic eye disease from retinal images, reducing the workload of human graders.
In the Early Detection and Diagnosis silo, we have the Community Acquired Pneumonia and Covid-19 AI Predictive Engine (CAPE), a collaboration between IHiS and Changi General Hospital. CAPE is used to predict the severity of pneumonia in patients from chest x-rays and enables clinicians to intervene early through timely triaging and treatment. In the Treatment & Disease Management silo, AI is being used in genomic analysis for precision medicine and protein folding simulation.
In general, there is a great expectation for AI to transform our healthcare system. However, I think that the current best state-of-the art AI is still far from human intelligence. In other words, it still lacks reasoning. Last year, I came across an interesting book titled “The Enigma of Reason.” It got me thinking that reasoning is an accumulation of experience and an aggregation of observations. Perhaps the next phase of AI is to augment different types of AI together to give a common perception of how the world works. For example, an AI solution for lung problems can be combined with an AI solution for heart conditions. Hence an AI with the capacity for reasoning would be able to draw generalisable inferences appropriate to different situations.
So, in its current state, it would still be best to augment AI with healthcare professionals to achieve “superhuman” intelligence. Use the computational power of AI to give faster results and use the meticulous nature of AI to pick up data details that may otherwise be missed by humans for swifter and safer patient care.
What are your priorities for the post-pandemic era?
In the new norm, I believe we will continue to find areas where AI can make the most impact on healthcare, such as AI decision support tools and remote monitoring of patients to be embedded in healthcare services.
Hence on a personal front, I set my priorities towards Machine Learning Operations (MLOps), the process of taking an experimental machine learning model into a real-world system. While Covid-19 has pushed healthcare systems to the edge, the pandemic has also paved the way for many innovative AI applications. To ensure that the good innovative AI work of many healthcare colleagues brings sustainable benefits, there is much work to be done. A few areas of focus include building consistent and good quality data pipelines, using AI to automate harmonisation of data, federated and continuous learning and Explainable AI (XAI).
Could you tell us a bit more about how IHiS is implementing AI in specific projects such as the Multiple Readmissions Prevention Predictive Model project, to manage diagnostics, admissions and re-admissions, and other healthcare challenges?
I will cover three projects demonstrating how IHiS has implemented AI:
- Diagnostics: Community Acquired Pneumonia and COVID-19 AI Predictive Engine (CAPE) supports efficient diagnosis of pneumonia patients
- Admissions: Tan Tock Seng Hospital’s Control, Command and Communications (C3) system helps support hospital operations including admissions and resource management
- Readmissions: Multiple Readmissions Prevention Predictive Model reduces the risk of patient readmissions
The multidisciplinary team includes:
- Clinicians who serve as subject matter experts on applying AI techniques in a clinical workflow.
- Health Informatics staff provide expertise in clinical information systems and decision support tools in hospital settings.
- Data Architects, Engineers and Scientists develop the machine learning algorithm, design the system architecture, implement the data pipeline and set up the technology infrastructure to run the AI solution
- Deployment Strategists (e.g. Operations and Delivery) provide ground implementation services and feedback in operating the AI solution.
- Business Translators (e.g. Policymakers and Administrators) assess and evaluate the value impact of the AI solution on the healthcare system.
Elsewhere, to support hospital operations, Tan Tock Seng Hospital’s Command, Control and Communications (C3) system provides real-time visualisation of the hospital’s ground operations. From the moment patients are admitted until they are discharged, C3 keeps track of resources such as hospital workers, beds, critical equipment, supplies and more. The system leverages AI to predict potential choke points and identifies the most optimal resolution approach to ensure patients are discharged in a timely manner. Video analytics and image recognition is also used to support crowd control. With the potential to be scaled nationwide, C3 can better support load balancing of healthcare resources across the island and allow for better coordination during a national crisis.
Finally, we know that prevention is better than a cure. The Multiple Readmission Predictive Model identifies high-risk patients for better care intervention to reduce their risk of being readmitted to hospital. This AI model analyses multiple facets of a patient via hundreds of indicators, and has an accuracy of 7 in 10 patients correctly predicted.
How did your interest and passion for data analytics first start? How has both the landscape, and your passion developed since then?
My PhD dissertation is in the use of mobile applications for industry control systems. In my academic days, I had always been intrigued in using mathematical formulas like regression to predict the mechanical engine’s time to failure. Further on in my career, I had seen the rise of Big Data and the benefits it can bring to the real world in evidence-based decision-making for businesses to optimise resources and impact. Much like an engineering control system, Big data provides a closed-loop feedback mechanism to measure the effectiveness of business decisions. Believing that I can put good use of my engineering knowledge in big data processing, I decided to specialise in data analytics, starting with visualisation and descriptive insights to machine learning and deep learning.
In my view, the change in landscape has been iterative. There has been a shift from having better infrastructure (e.g. NoSQl) in the beginning to enhancement of analytics techniques. Now, the cycle has swung back to ensuring a good quality data pipeline. What this change probably means to the AI practitioner is that there are always more things to learn.
As for my passion, I am always fascinated by the different types of data and its use cases. I have considered myself fortunate to have made the decision to join the HealthTech industry 6 years ago. Not only is there a myriad of data, there are endless possibilities for data innovation in services, physical devices, processes and planning. I would think it is a pilgrimage that a data person should take at least once in their career.
If there is any change in my passion, I would say that initially data to me was just hard numbers used to improve processes. Since I joined the healthcare industry I understand that data has a great impact on the life of a patient and their family.
How do you feel the healthcare industry compares to other industries when it comes to AI implementation? What specific challenges/opportunities does it meet?
The use of AI in healthcare has its strengths and weaknesses. One advantage of Health AI is that its development process and required trials tend to be more robust than AI in other industries, providing safe, efficient solutions for frontliners and patient care. The downside of Health AI is that it tends to take a much longer time to implement. But this is not always the case, as I’ll explain below.
Because of our different professions and experience, healthcare providers look at problems from multiple perspectives. Diverse perspectives and approaches are good for AI, making it inclusive and fair. But it will also mean that we must cater for different professional views and good change management strategies. In addition, Healthcare AI practitioners need to adhere to the principle of nonmaleficence due to the potential risks to patient safety, especially for clinical AI. As such the use of AI in healthcare understandably requires closer scrutiny.
As a result, compared to other industries which have deployed AI, the use of clinical AI in healthcare will take a longer time to implement in consideration of patient safety, but it will also mean Healthcare AI is more robust and seeks to bring the best benefits to the patient.
To accelerate the long development time required for Healthcare AI, IHiS proactively seeks out clinicians that have viable problem statements to co-create with them in a start-small scale-fast approach through Minimum Viable Product (MVP) iteration and Proof of Value piloting.
Not all Health AI applications need a long development and implementation timeline. There are also non-clinical Health AI applications in the areas of administration and operations which can be implemented relatively quickly. For example, the Command, Control and Communications (C3) system at Tan Tock Seng Hospital was deployed in about four months as a rapid response to the Covid-19 crisis. IHiS was able to quickly develop and implement additional features to the system, which uses AI and data analytics to help healthcare workers manage resources more efficiently.
Instead of seeing AI as a potential challenge, how do you think healthcare professionals work alongside AI technologies to develop better understanding and analysis of healthcare data?
We frequently hear professionals asking when AI will replace the first radiologist. When I had the opportunity to work with some local senior radiologists, out of curiosity I asked them whether they fear AI taking away their job. The common answer I receive is “no.” In fact, AI to them is a smart companion that pre-analyses radiology images, highlights the presence of abnormalities and is a good helper in prioritising reporting worklists.
I sense that locally most clinicians do not adversely view AI as a challenge. On the contrary, there are many who are quite ready to embrace AI. If there is anything that holds clinicians back, it is the “black box” phenomenon of AI algorithms. It is understandable, because in healthcare we are dealing with patient’s lives and well-being, therefore clinicians supported by AI must know how it works. As such it is important for the data scientist working on AI solutions to factor explainability as part of the development. For healthcare professionals, they will need to rethink how they can work with this AI companion to achieve better care outcomes for the patient.
How can AI help Singapore further develop and establish itself as a global leader in healthcare innovation?
Singapore aspires to be the 'living lab' to productionalise global AI solutions. We have good regulation and processes, good coordination and synergies between various government bodies to drive AI, and a highly digitally literate population that embraces new technologies.
In terms of healthcare, I think that we have more to offer to the world. We have a multi ethnic population that can validate AI solutions’ generalisability, a healthcare system that is world-class, a National Electronic Healthcare Record (NEHR) that enables longitudinal study of the patient journey, and much more. In short, Singapore is well positioned to establish an end-to-end Healthcare AI ecosystem; impactful clinical use cases, data readiness, AI development and real-world validation.
Interview responses have been edited for clarity.
Want to find out more about IHiS’s work in AI? Catch up on their session from AI x GOV here!